
To SNAP or not to SNAP: Evaluating Pharmacy Dispense Data for Safety Net Antibiotic Prescriptions (SNAPs) in Pediatric Acute Otitis Media with a Large Language Model
0
0
0
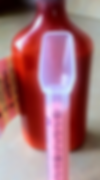
Acute otitis media (AOM), commonly known as an ear infection, is the most frequent reason for antibiotic prescriptions among children. Yet, a significant portion of these cases resolve on their own without the need for medication. Overprescription of antibiotics is a known issue, contributing to antibiotic resistance, a severe threat to global public health.
To address this, Safety Net Antibiotic Prescriptions (SNAPs) are recommended. These prescriptions are given with the advice that parents delay filling them to see if symptoms improve, thereby reducing unnecessary antibiotic use. However, tracking these prescriptions is challenging due to their absence from structured clinical data.
Recognizing the potential of advanced technology to tackle this problem, UCSF Health embarked on a pioneering study to utilize artificial intelligence, specifically large language models (LLMs), to identify SNAPs within unstructured physician notes. This approach could significantly enhance antibiotic stewardship programs by providing a scalable and efficient tool for monitoring and potentially reducing antibiotic overuse in pediatric care.
The Study
Our study employed UCSF’s Versa, a PHI-safe, HIPAA-compliant deployment of the GPT-4o model, to analyze pediatric AOM treatment plans from free-text physician notes. We explored various methodologies: zero-shot, zero-shot with guardrails, and few-shot classification, alongside a locally trained model, Clinical LongFormer, to determine the most effective strategy for identifying SNAPs.
Results
We analyzed 5,707 pediatric encounters for AOM, manually reviewing 374 notes for validation. The zero-shot approach using Versa achieved an impressive 97.8% classification accuracy, outperforming other methods. The Clinical LongFormer, a smaller and more cost-efficient model, also showed promising results with 91% accuracy.
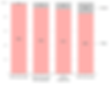
These findings are encouraging as they demonstrate that LLMs can effectively identify treatment plans, including SNAPs, from clinical notes with high accuracy. This capability presents a significant advancement in pediatric antibiotic stewardship, offering a scalable solution to monitor and potentially reduce unnecessary antibiotic use.
Implications
The successful implementation of this AI-driven approach could serve as a model for other institutions, enhancing antibiotic stewardship efforts across the healthcare system. Furthermore, this technology could be adapted to other areas of healthcare, where understanding treatment plans from free-text notes could drive quality improvement and cost-efficiency.
Conclusion
This study underscores the substantial potential of leveraging LLMs to enhance antibiotic stewardship. By effectively categorizing treatment plans from free-text physician notes, we can provide a valuable tool for healthcare providers to track and optimize antibiotic usage, ultimately protecting our youngest patients from unnecessary treatments and contributing to the fight against antibiotic resistance.
UCSF’s commitment to integrating innovative technologies like Versa into clinical practice not only improves patient care but also sets a standard for the future of healthcare operations, emphasizing the critical role of AI in advancing medical treatment and management strategies.

By Jessica Pourian, MD
Pediatrician, Informatics Fellow
University of California, San Francisco
ACIF President